Texas State engineering gets $250,000 grant to improve pavement condition data collection
Julie Cooper | August 7, 2022
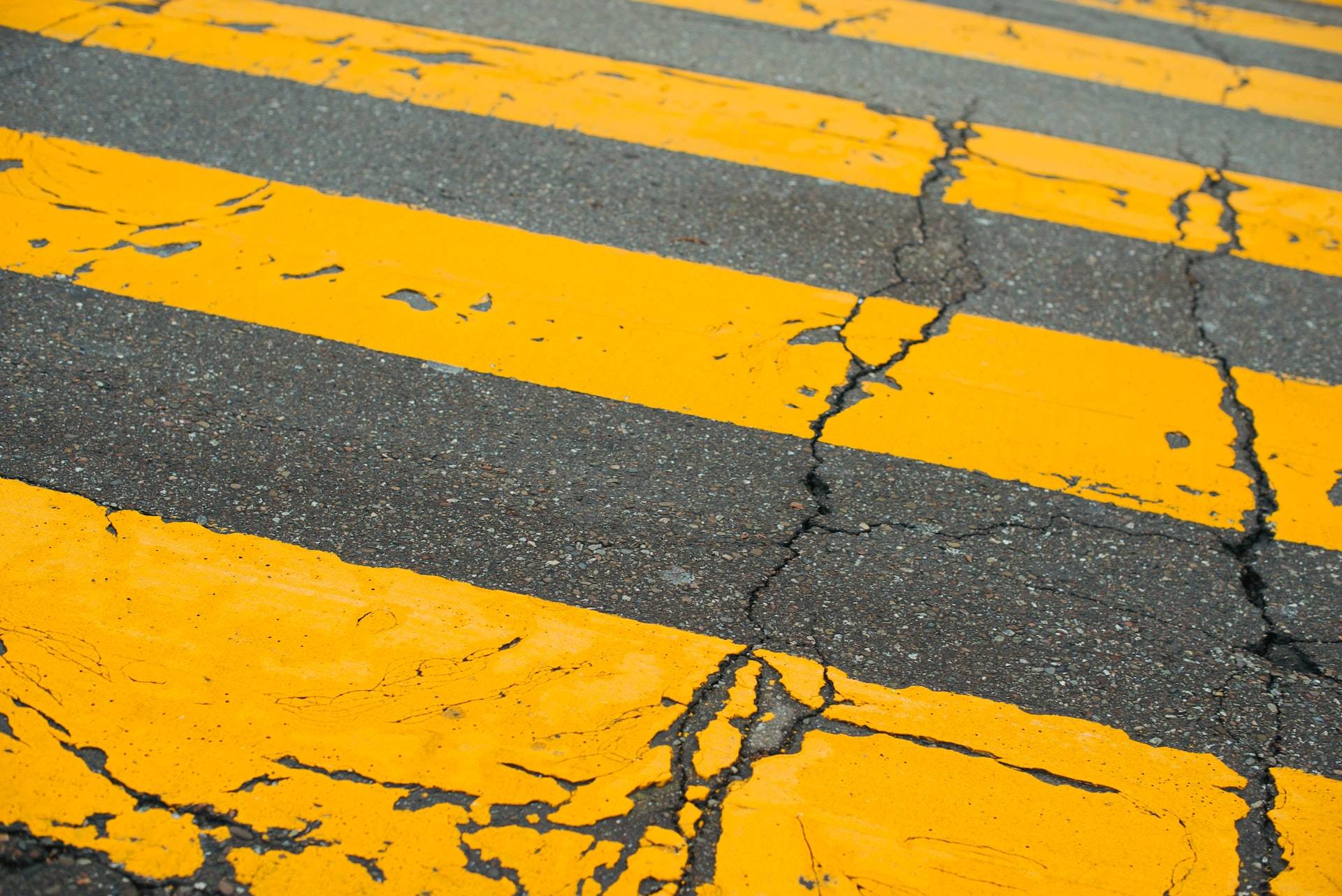
Texas State University has received a $250,000 grant from the National Science Foundation (NSF) for a research project titled, “Using artificial intelligence to improve the accuracy of automated pavement condition data collection.”
The two-year NSF grant will use machine learning to detect and measure the cracking distresses on roads in the U.S., with the aim for better use of budgeting and technology for Pavement Management Systems.
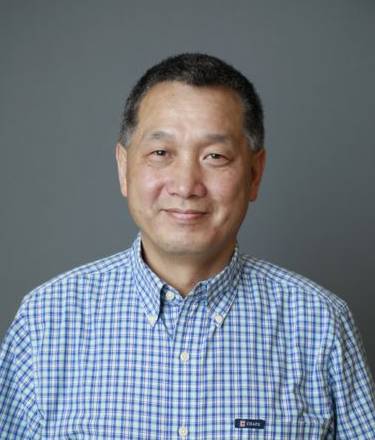
Feng Wang, associate professor in civil engineering of the Ingram School of Engineering (ISoE), is the principal investigator (PI) of the project. He received his Ph.D. in transportation engineering from The University of Texas at Austin and has conducted research, teaching, and service in the field for more than 20 years. His current research activities include automated pavement condition data collection technologies, pavement condition data analysis, and decision support modeling for pavement management systems and pavement warranty program.
The broader impact/commercial potential of this Partnerships for Innovation -- Technology Translation (PFI-TT) project is in enabling pavement engineers to make sound maintenance decisions through a novel, cost-effective, accurate and reliable automated pavement condition data collection system. The proposed technology should reduce the costs and improve the quality of pavement data collection. A successful application of the new technology is expected to significantly benefit society by improving the maintenance of transportation infrastructure maintenance and, subsequently, other infrastructure systems. A major societal contribution of this project is the acquisition, accession, management, and public sharing of pavement distress images using industrial standards.
Sharing the image data generated under this PFI project will encourage additional research and competition in the field, which will ultimately improve pavement image processing abilities. Furthermore, this project will provide entrepreneurial education and leadership development opportunities for a postdoctoral researcher, and graduate and undergraduate students. The recent inclusion of an automated pavement condition data collection system of industry-standard to the lab inventory of the Ingram School of Engineering has provided strong support to the NSF project.
“We support our postdoc and Ph.D. students who are working very diligently on this proposal,” Wang said. Xiaohua (Nemo) Luo, is the postdoc research associate and Co-PI, along with doctoral students Haitao Gong and Jueqiang Tao, of the Materials Science, Engineering and Commercialization (MSEC) program in the College of Science and Engineering. Haitao has made a significant contribution to the success of the NSF PFI proposal. Haitao’s Ph.D. dissertation research titled “Improving Pavement Distress Detection with Deep Learning” has served as the foundation of this NSF project.
To overcome the inaccuracy and reduce the costs associated with existing methods, the proposed technology will use machine learning-based image processing algorithms, which are more promising in handling the heterogeneity of pavement characteristics than the traditional distress detection methods. In addition, the proposed system is expected to improve data quality assurance by using analytical capabilities to identify problematic data and reduce errors.
Wang said it has only been in the last decade that technology including sensors and cameras have been utilized to evaluate road conditions. The majority of states have adopted the automatic data collection for their pavement management — but there are poor quality issues with this automatic data collection. The goal is to use machine learning to make image processing smarter.
Finally, the proposed system is expected to reduce the use of costly hardware, thereby increasing the financial sustainability of pavement and infrastructure management. The core components of this system are cost-effective 3D image acquisition, machine learning-based pavement image processing algorithms, and systemic design and optimization-driven data quality assurance.
“We can work together with other researchers -- with computer science and other engineering programs or other universities, each contributing our strengths to make the technology better,” Wang said.
Share this article
For more information, contact University Communications:Jayme Blaschke, 512-245-2555 Sandy Pantlik, 512-245-2922 |